Podcast | Healthcare (HC)
How Generative AI Is Reshaping Healthcare
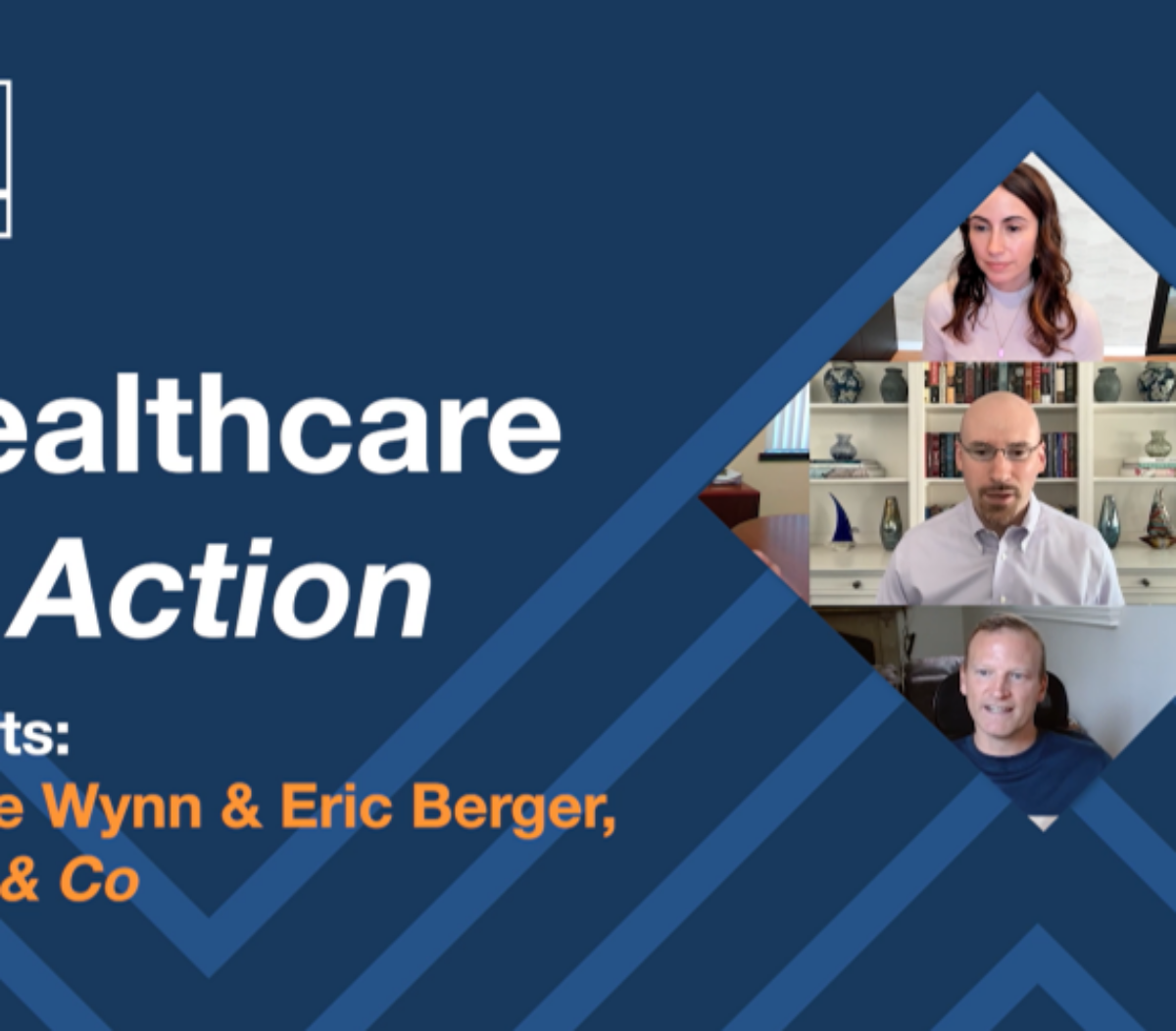
THL’s Healthcare in Action, Episode 5
Generative AI has exploded into the healthcare space, but where is it actually creating value, and where is it just noise?
In this episode, hosts Jon Lange and Josh Nelson are joined by Grace Wynn and Eric Berger, partners in the healthcare and life sciences practice at Bain & Company. Together, they explore the evolution of generative AI, why it has taken center stage, and how it’s starting to impact health systems, clinicians, payors, and patients alike.
From ambient listening to automated claim letters, this episode cuts through the hype to highlight real-world applications (and unintended consequences) of GenAI in healthcare.
Key Takeaways:
- Use cases in healthcare are growing fast, especially in administrative tasks like medical documentation, prior authorization, and patient communications.
- AI’s impact on clinicians is most promising where it reduces friction without removing human oversight.
- The payer-provider dynamic is becoming a “bot-on-bot” arms race, with both sides automating increasingly complex workflows.
- Patients will likely first feel the impact of GenAI through AI-powered chat tools that streamline scheduling, pre-appointment instructions, and multilingual support.
[02:22:28] Eric Berger AI, artificial intelligence, is not really a new technology domain by any means. It’s been around arguably since World War II.
[02:22:36] Grace Wynn I think, it’s a bit, like, saying adopting a PC in the 80s is a competitive advantage. Yes, it’s good to be early and allows you to be ahead of the time, but eventually I think everyone is going to need to get there too.
[02:22:48] Josh That’s Grace Wynn and Eric Berger. They’re partners at Bain & Company. And I’m Josh Nelson, head of healthcare at THL Partners. And this is Healthcare in Action. It’s a podcast that explores the latest developments and innovations transforming the U.S. Healthcare sector, from cutting edge technology to thoughtful approaches to patient care. I’m here with my colleague, Jon Lange, who’s going to lead us in a conversation about GenAI in healthcare, including the applications that are already taking shape, the challenges of adoption, and the many exciting opportunities that lie ahead. Jon, take it away. Thanks, Josh.
[02:23:27] Jon Seems like AI comes up in every conversation these days, and that’s certainly true in healthcare, where it has the potential to drive significant advancements and transform the landscape. Healthcare organizations have faced mounting pressures in recent years, from labor shortages, to rising costs, to outdated infrastructure. AI and other innovations have the potential to make a big impact in addressing these challenges. Take payers for example. According to a recent Bain & Company survey, payer organizations are prioritizing IT investments in care coordination, utilization management, and claims processing. These investments can help identify high-risk members, streamline care delivery across providers, and ensure accurate and cost-effective adjudication of claims. Yet challenges persist. Over 65 percent of payers report struggling with legacy technology that hampers scalability, flexibility, and cost effectiveness. Recently, generative AI has been gaining attention as a potential solution to all these challenges. Both payers and providers are piloting AI-powered solutions to improve operational efficiency, optimize resource allocation, and enhance member and patient experience. But a variety of barriers still exist, including regulatory concerns, implementation costs, and the accuracy of AI solutions. To fully unlock AI’s potential, the industry is going to need to address these concerns while fostering transparency, collaboration, and ethical governance. On today’s episode, I’m joined by Grace Wynn and Eric Berger, partners in the healthcare and private equity practices at Bain & Company. They’ll share their insights on how AI is shaping the future of healthcare, and what it all means for patients, providers, payers, and the U.S. Healthcare system overall. So let’s get into it. Grace and Eric, thank you for joining me.
[02:25:09] Eric Berger Thanks for having us, Jon.
[02:25:10] Jon So, of course, I’m sure most people watching this are very familiar with Bain & Company. But, Grace, would you mind starting out just talking a bit about what you and Eric do in your practice?
[02:25:21] Grace Wynn Thrilled to be here, Jon. So Eric and I, as you said, are both partners in our healthcare and life sciences practice. We spend all of our time working across the healthcare sector with investors, with our portfolio companies, thinking about how to drive sustainable, durable growth. And obviously over the last 18 months, GenAI has been a critical part of these engagements.
[02:25:43] Jon I think that is a good starting point. Generative AI seems to have come along so quickly. Can you just put it in a little bit of context, maybe a little of the history, how has AI evolved in the past several years? How did we get to where we are today with GenAI being on everybody’s minds?
[02:26:01] Eric Berger So AI, artificial intelligence, is not really a new technology domain by any means. It’s been around arguably since World War II. Artificial intelligence and machine learning generally refer to a set of analytical techniques through computer science to solve a variety of problems, and there’s different mathematical, you know, algorithms and techniques that sit inside of those programs. What is new and different about GenAI is the following. So in 2017, I believe, Google published a paper that’s generally referred to as the transformer paper. It is transformer is the T in ChatGPT and all of the others. And so what happened was it’s a different kind of mathematical model that’s really, you know, based on predicting the next best letter, the next that’s pixel, the next best thing, you know having understood and digested everything else. So at its core, it’s it’s, a probabilistic model. It is taking a set of probabilities around, you know what is the letter that comes after this letter, given the prompts you’ve written and everything ever written in any language ever to begin to say what is the probability that the H comes after T and E comes after H, therefore, it’s a thought. And being able to tweak that and use that, you know, translate that into mathematics is essentially what the transformer models do in an overly simplistic way. And so this technology specific to what we’re calling kind of GenAI or foundation models comes back to a set of transformers that are arguably six or seven years old. And so what we’ve seen is the explosion of progress being made using these transformer models with respect to text, voice, image, and kind of reasoning and judgment, and that’s been kind of where this has happened. If you think not about the last six, seven years but about the last one to two, what happened when GPT-3 was released was essentially 3 was such a major step function improvement from the prior models at 2. That it actually kind of exploded into the consciousness of everybody talking and thinking about it and kind of why. The difference between 2 and 3 was kind of these models looking like a science experiment, where they’re kind of interesting but not useful because there’s so much, there’s still enough gibberish and illogical stuff that gets spit out, that it’s just not all of that useful for any like real life application. By the time you get to 3 or 3.5, the output of the models is so astonishingly good that it starts to look and feel like responses you would get, you know, colloquially from a high school student, not a Nobel Prize winner, but like a high-school student. And so it looks pretty darn good. So that was like two years ago. And now we’re at the mode of kind of anticipating the next step function up. The question with the next wave of models is going to be, are they at or above graduate school, like PhD level, quote unquote, intelligence or capability? There’s a lot of excitement about like what those are gonna look like going forward.
[02:29:21] Jon Great, and I think that frames it up really well as we focus on healthcare. And so of course, generative AI is applicable across all industries, but we all focus on healthcare, and there are so many potential applications here. Can you just frame up for us how you think about the key challenges in healthcare that AI could address and the opportunities for AI to be helpful?
[02:29:44] Grace Wynn In terms of what we’re seeing today, two ways I would frame that. One is what use cases are getting a lot of traction today, and then two, where can we actually quantify impact already? On number one, there are enormous amount of use cases getting traction across, as you said it, every sector of healthcare. Within pharma and med tech, you’re seeing field team enablement, regulatory writing, process automation, just to name a very small subset. Within payer, there’s cost containment, like prior off-claims and outs and appears, as well as member engagement, like automating your call center interactions, your care management services. And then within provider, just to delve into this one in a bit more detail, if we break this into administrative and clinical, for administrative functions, there’s a number of exciting areas. The theme around them, and this is really important, is that the use cases improve provider efficiency without eliminating the need for that clinician. And so four categories, just to name a couple that are gaining traction and not at all holistic, but one is this ambient listening, speech-to-text medical documentation. The second one, automated drafts to patient engagement that’s really helping lower clinician time writing these. The third piece around medical writing assistance, so using drafts for post-procedure instruction, prior auth, letters to payers, appeals, denials. And then the last one is interesting, it’s call center routing. One health system implemented this and they cited their abandonment when you call in, went from 64 to 27 percent, so pretty meaningful improvement. I think we’re still early days in terms of, and so we’re focusing more on leading indicators that show how you will create significant value for the organization. And so you’re seeing more metrics like internal employee adoption of tools, patient experience, clinician reported speed, accuracy, satisfaction, but I think you’re gonna see more of the further quantification over the next year or two.
[02:31:50] Jon Got it. And so I think you very thoughtfully laid out different subsectors within healthcare in different end markets, provider, payer, pharma, et cetera. Within provider, I think you laid it out in an interesting way, sort of the dichotomy between administrative and clinical. And I guess, how do you think about the relative applicability of GenAI today in administrative versus clinical.
[02:32:16] Eric Berger We were just, you know, had the benefit of listening to Epic and other EHRs talk a little bit about their plans, and you know, just the massive amount of administrative work that happens between a doctor or a nurse or an administrator and either the EMR record or other software tools is enormous. And so to the extent this technology can lower that burden by predicting or at least accelerating the documentation. And I mean, I think, you know what, Abridge and Nuance or Dax have done in terms of ambient listening, converting an almost real time, close to real time of physician visit based on the verbal cues that one observes into a note, which then gets populated into a claim, which can then get into a prior auth letter. I think we’re just going to see massive acceleration of some of that. I think that the question as to whether or not that actually leads to results is the payers are going to do the same thing. And so you have this kind of yin and yang, or this, like, intergalactic battle between both sides, kind of automating administrative work. And so, you’re gonna have bot on bot conflict, which is what we already see, right, in call centers with prior off. I think the challenge is going to be, you know, where does that prompt kind of a rethinking of some of the technology workflows and candidly like the pipes between providers and payers to try to eliminate or reduce that work, as opposed to just automate escalating levels of burden. One of my favorite uses, I heard a physician talk about using ChatGPT to write a prior authletter, but the typical prior off-letter was like two paragraphs. He was so fed up with this given payers record of denials, you know, he essentially told ChatGPT loaded a bunch of articles and was like, give me the 34 page version of this letter, just to put gunk in the wheels and prevent the automatic denial from coming through. So anyway, the technology can be used for good.
[02:34:14] Jon It’s a really interesting point. And of course, any technology has pros and cons, as you say, it could be used for good or evil. I wanna come back to that payer-provider conflict or set of incentives. But before we get there, from the patient perspective, how are patients going to experience the impact of generative AI in the near term, in the next one or two years? And is that gonna be a positive impact to experience or a negative one?
[02:34:43] Eric Berger I imagine it’s too early to tell. I mean, it’s like any kind of technology adoption relative to what people are accustomed to. You know, the ATM is a much more streamlined way to go get cash out of the bank without a human in the process. Similarly, you can imagine for scheduling, for answering question, routine questions, like, I have a colonoscopy tomorrow, what is my prep? And having someone explain that to you. You can ask questions about it. You know, things that are relatively straight forward with low downside risk people can be interacting with a GenAI enable chatbot, and I mean, I think, their carries a lot of promise here, you know, that chatbot can switch language instantly, and not just the six or seven most commonly used languages but any language in the world. That chatbot cannot adjust the level of reading and comprehension that the type of language that it uses up or down, so you can describe how to do certain things at a third grade reading level, at a 12th grade reading, level in Shakespeare and, you know, iambic pentameter. You can adjust all of that instantly with no cost. And so that has a lot of opportunity to increase access, streamline communications, and so forth. I think, again, I’ll just give you the counter there is as you do that. People are worried about fraud, people are worried about, you know, poor use, people can pretend to be your doctor, people can be all sorts of things.
[02:36:10] Jon You know, as a consumer of the healthcare system, of course, we’re all consumers of the healthcare system. Clinical improvements sound quite exciting to patients. And so, again, from the patient perspective or from the provider perspective, what clinical advances should people be most excited about as a result of AI.
[02:36:30] Grace Wynn I would say cell imaging. AI can detect cancer more consistently than the human eye. And so there’s a question of what does this mean for the future of care, for the democratization of care? Will everyone have access to the leading way to diagnose breast cancer, for example? And I think there’s great example even now of the early innings of that. There’s a professor at Mass General Brigham. That’s been doing cutting edge work on applying AI to cell images, and then turning that into a tool for pathologists. This could meaningfully increase the standard of care, and it doesn’t eliminate the need for the pathologists, but it lowers the work versus what is required today. And I think if you think about where AI could be in the biggest impact in the coming decades, I think the democratization of healthcare through AI is possible.
[02:37:24] Jon It’s really exciting. And Eric, any other examples you’d add??
[02:37:27] Eric Berger So we see, to Grace’s point, a huge momentum in radiology and applications that the medical OEMs are deploying, so GE Healthcare and others, so we see more interest in momentum in pathology, which is where Grace mentioned. And so there’s a variety of startups and pretty interesting ideas happening. I think kind of the broad category, and it’s not GenAI per se, but it’s the next generation of the alpha fold models. So those are Google’s models that again, you know, you’ll hear the recurring refrain, it is predicting the shape of how a three-dimensional structure of a protein, again, thinking sequentially about how this thing is going to interact through a variety forces. You know, we are seeing more and more examples of using AlphaFold and some of their new derivative models with really impressive results for discovery and applications within life science.
[02:38:23] Jon Got it. And it would be great if you wouldn’t mind just giving a little context on AlphaFold, and what that means, where we were before, where this is going. One example I heard recently is that one of those projects determining one protein fold, not so long ago, took sort of the effort and the time of an entire graduate student thesis. And suddenly with this new technology, we’re several orders of magnitude more efficient in understanding those folds, and that could dramatically accelerate discovery, but can you spend a minute on that?
[02:38:59] Eric Berger I mean, the way the world used to work is you would spend your PhD in your 20s, essentially figuring out the right way to crystallize a protein. And so you crystallize it by putting it in some suspension, cooling it down, and getting it to freeze, then you bump a lot of light imaging through it, you see the refraction of that light, and then you can infer what the shape of that protein looks like when it’s frozen. And then from the shape of that protein, you can begin to think about either a small molecule or monoclonal antibody or a protein binding to some site in that protein that changes either its function, its shape, etc. That was like traditional drug discovery, which is, you know, you would do structure-activity-based drug design, you would wait for certain proteins to get crystallized so you could imagine what would fit in the binding pocket of a given thing and kind of gunk up the works. Today, and again, it’s not perfect, but you can start to imagine, first they started predicting the shape, now they’re predicting the binding properties, kind of in silico binding properties of something to something else. And to lock in the key analogy, instead of both being actual chemical entities that you measure binding constants for, now you’re doing it on a computer and doing that. And so the magnitude of how far we’ve come in 20 years is astounding.
[02:40:22] Jon And can you maybe take a step back on that, just frame up for us, what does that mean? Where were we 20 years ago in terms of the drug development, life cycle, timing, and what could this mean going forward?
[02:40:34] Eric Berger I think, it’s too early to tell, I mean, you know, it used to historically, it does continue to take, you know a long time to develop a new drug. And there were all of these classes of, you know undruggable targets is what you would call them. And so, you now can you unlock some of the undruggable targets by having better predictive powers from AI to kind of cut the time or cut the magnitude of investment required to identify a drug candidate. Maybe. You know, I think we’ll be closer to knowing the answer to that in five to 10 years. The theory of the case, which is if you can create some technologies to do that, and let’s say in parallel, you start creating PhD-level quote, intelligence on kind of the basic research side of it, and you begin to pair those together, you can unlock faster and better drug development. That is the hypothesis we will see.
[02:41:26] Jon Grace, maybe coming back to something you said earlier, we’ve talked a lot about some of the applications we’re already seeing across provider, payer, pharma, med tech. Where do you see the impact of AI in 10 or 20 years? What are the capabilities AI will have then? And what are you most excited about?
[02:41:46] Grace Wynn There’s two important points to make on this, on where we see GenAI in 10 to 20 years. One is Amara’s law is absolute revel in here. Humans have a tendency to overestimate the impact of technology in the short term, but in the long term, we all tend to underestimate the impact. And then two, as you look across industries for a second, Bain anticipates healthcare, life sciences, and financial services are the sectors that are going to be most disrupted by AI over the next 10 years. And it’s quite telling that pharma companies, to Eric’s point, have jumped on GenAI so early, because of how deeply disruptive it can be to their core business. So like as we’ve talked about massively increasing the number of targets in clinical applications, yield of molecules to actual drugs and reducing that clinical development timeframe from five to seven years to 20 to 30 percent lower or whatever ends up being. And then within provider, I think you’ll see massive improvements in clinical decision-making, and I think, you’ll massive productivity transformations for clinicians as well. But what you won’t see is an elimination of the need for clinicians.
[02:42:57] Jon Maybe switching gears a little bit. Of course, at THL, whether we’re investing in healthcare IT companies or healthcare services companies, we’re always thinking about the impact of AI on those companies, on their competitive dynamic, on their ability to innovate. As you think about maintaining or extending a company’s competitive advantage, what do you look out for in terms of leveraging AI and also protecting against competitors using AI?
[02:43:26] Grace Wynn I think first, you need to figure out what your ambition is with AI. Is it keeping up with the Joneses? Is it being a fast follower? Is it actually being a leader or disruptor? If you’re in bucket one or two, even if you aren’t striving to have a competitive advantage in GenAI, every business is going to need to evolve its model, because of how pervasive the impact is going to be over the long term. If you’re in number three, and you’re actually aspiring to be a leader, a disruptor in GenAI, and that’s a part of your competitive advantage, it will be less expensive. It also will mean the company will spend a lot of time on trial and error, and others will then be inspired by it, fast copy it, and soon may have a similar model to it. I think it’s a bit like saying, aadopting a PC in the 80s is a competitive advantage. Yes, it’s good to be early and allows you to be ahead of the time, but eventually I think everyone is going to need to get there too. And I think it’s not so dissimilar from e-commerce as well, where companies jumped onto e-commerce first, invested heavily. Some of them grew faster as a result, some of them did not. And you saw others wait it out, figure out how to compete against Amazon, and they’re still around. And I think the important point is GenAI is on a path to being ubiquitous. It’s obviously not there yet, right? We’ve got a long way to go. But eventually every offering, every customer experience will have an AI aspect embedded into it. And it’s just going to be part of the normal operations. And I that’s the important points for these companies.
[02:44:57] Jon Got it. And so maybe to say that a different way, or to summarize, it sounds like it is quite important, maybe even mission critical for really every company to think through AI and have an AI strategy. But at the same time, your view is that that isn’t going to give you a sustainable advantage. Maybe there’s a relatively short first mover advantage, but that that will go away relatively quickly. Is that fair?
[02:45:20] Eric Berger I think, well, the basic technology is going to be broadly available via closed models and open models. So that access to those models is not necessarily a source of advantage. The source of competitive advantage is going one come from the data that you’re using or the workflows you build on top. And so kind of your ability to do either of those two things and build a muscle around it will become how you can think about competitive advantage. But the technology itself and the access to it is just going to be access to other technologies like cloud services. Right? So, you know, there’s a world in which we begin to think about GenAI like the cloud. And so it helps you save money. It helps you store your data effectively. You have choices, public or private. Is the cloud a source of competitive advantage for people? No. But what people do with it, whether it’s using it to lower your cost position, use it to streamline your entire tech stack, et cetera, et cetera, that becomes kind of where you can begin to separate.
[02:46:27] Jon Eric, some of our listeners are young professionals, maybe getting out of college or getting out a business school. What advice would you give them embarking on a career in consulting and particularly in healthcare consulting?
[02:46:40] Eric Berger So the advice I give is to follow what you’re interested in, and show some determination and perseverance as you do it. And I mean, Grace and I have worked together for probably more than 10 years at this point. And, you know, we’ve done a lot of work together. That’s covered a lot of different topics, a lot of different clients, a lot of different circumstances. And I think, you know, one of the reasons I like working with Grace, but one of the reasons, I think it stood out to me that the qualities that I would say others should try to emulate, but just perseverance, commitment, intellectual curiosity, those are the kinds of things we look out for at Bain. But personally, I look out with the people I like to work with, the clients, Jon, like you and other folks at THL, I think, that tends to be a pretty universal set of characteristics that can, you know, lead one to be successful. Healthcare is complicated. It’s hard. It changes the nature of healthcare changes. And so unlike other businesses, not underestimating the amount of change in the other industries, but like there’s a lot of change, there’s lot of complexity. And so you have to kind of know that going in, and want to do it.
[02:47:50] Jon Great. Grace, any advice you’d give to someone coming out of college or coming out a business school who wants to get into consulting, or in particular consulting for healthcare companies?
[02:48:01] Grace Wynn I think, Eric said it really well. I think one piece of advice I got early on is that, I think for a lot of these industries, not just consulting, being smart is a little bit of table stakes. It’s having a growth mindset. It’s being gritty, where folks tend to both enjoy the job more and do well at it. And I would worry less about how much you know today, and worry more about kind of, are you taking a growth mind set to what you’re doing?
[02:48:24] Jon Grace and Eric, thank you so much for being with me today.
[02:48:27] Eric Berger Thanks for having us, Jon.
[02:48:31] Jon As we wrap this episode, I’m joined by my colleague, Shahab Vagefi, head of healthcare IT at THL. Shahab, as you reflect on Grace and Eric’s comments on AI in healthcare, how do you see use cases evolving over the next five to ten years?
[02:48:47] Shahab Jon, it’s such an exciting area, and one point that really resonated with me was how the AI adoption curve may progress at a different pace for administrative versus clinical use cases. We’re already at the point we’re bringing AI to bear to address manual administrative tasks can lead to powerful efficiencies, but we still have a long way to go before AI is sufficiently accurate and reliable to be a consistent substitute for human clinical decision making. There’s no doubt that clinically focused AI is advancing quickly. But as we think about the evolution of use cases in the next few years, I’d expect the impact on administrative tasks to be staggering in the near and medium term, while the impact of clinical use cases will take longer to develop. And from an investment perspective, that’s an important consideration as we thinking about new products and the potential disruption of existing businesses.
[02:49:37] Jon From an investment perspective, does AI impact the way you think about innovation within HCIT companies or their ability to sustain a competitive advantage?
[02:49:47] Shahab It’s a really interesting question, and it’s important to remember that in addition to having a major impact on end customers in the healthcare system, AI will absolutely have an impact on how HCIT companies innovate and compete. Grace and Eric talked about the potential for AI to make traditional modes narrower. And I think that goes back to something you discussed with Adrian Schauer, the founder and CEO of AlayaCare. Since AI is making it easier for technology companies to build features, HCIT will need to build competitive advantages in other ways. Whether that’s through proprietary IP, innovative business models, network effects, or other avenues that may not have been necessary before AI changed the healthcare landscape.
[02:50:28] Jon And taking a step back, there are just so many use cases across healthcare where AI could make an impact over the longterm. How do you think about AI’s impact for different stakeholders in different customer end markets, like providers, payers, pharma companies, and of course patients?
[02:50:46] Shahab Jon, you’re exactly right, that the sheer number of use cases is staggering. One of the really exciting things about AI is that even in its current state, it can go a long way to advancing healthcare and eliminating key pain points for just about everyone. For example, AI can help reduce paperwork for both providers and payers, and that has the potential to make a real impact on provider burnout, and allow providers to focus more on patient care. In the life sciences industry, in addition to administrative tasks, there’s a really interesting use cases around drug discovery that could substantially reduce the time it takes to develop new drugs. And all of this could ultimately make a big impact on patient experience and outcomes. So while of course, there are many important risks we have to be vigilant about, AI has the potential to be a very powerful force for good for just about every stakeholder in the ecosystem.
[02:51:37] Jon Shahab, thanks so much for joining me. Really appreciate your thoughts as always. And I’m looking forward to continuing the conversation with you, Grace and Eric very soon.
[02:51:46] Josh Thank you for listening to Healthcare in Action, brought to you by THL. To help Healthcare in Action, reach more listeners like you, either share this episode with a colleague, subscribe to the show, or rate and review us on Apple Podcasts. And for more background on THL’s Healthcare Vertical, visit thl.com/verticals/healthcare.
- Verticals
- Healthcare (HC)
- Verticals
- Healthcare (HC)