Podcast | Financial Technology & Services (FTS)
Revolutionizing Risk Assessment: Automation’s Role in Insurance
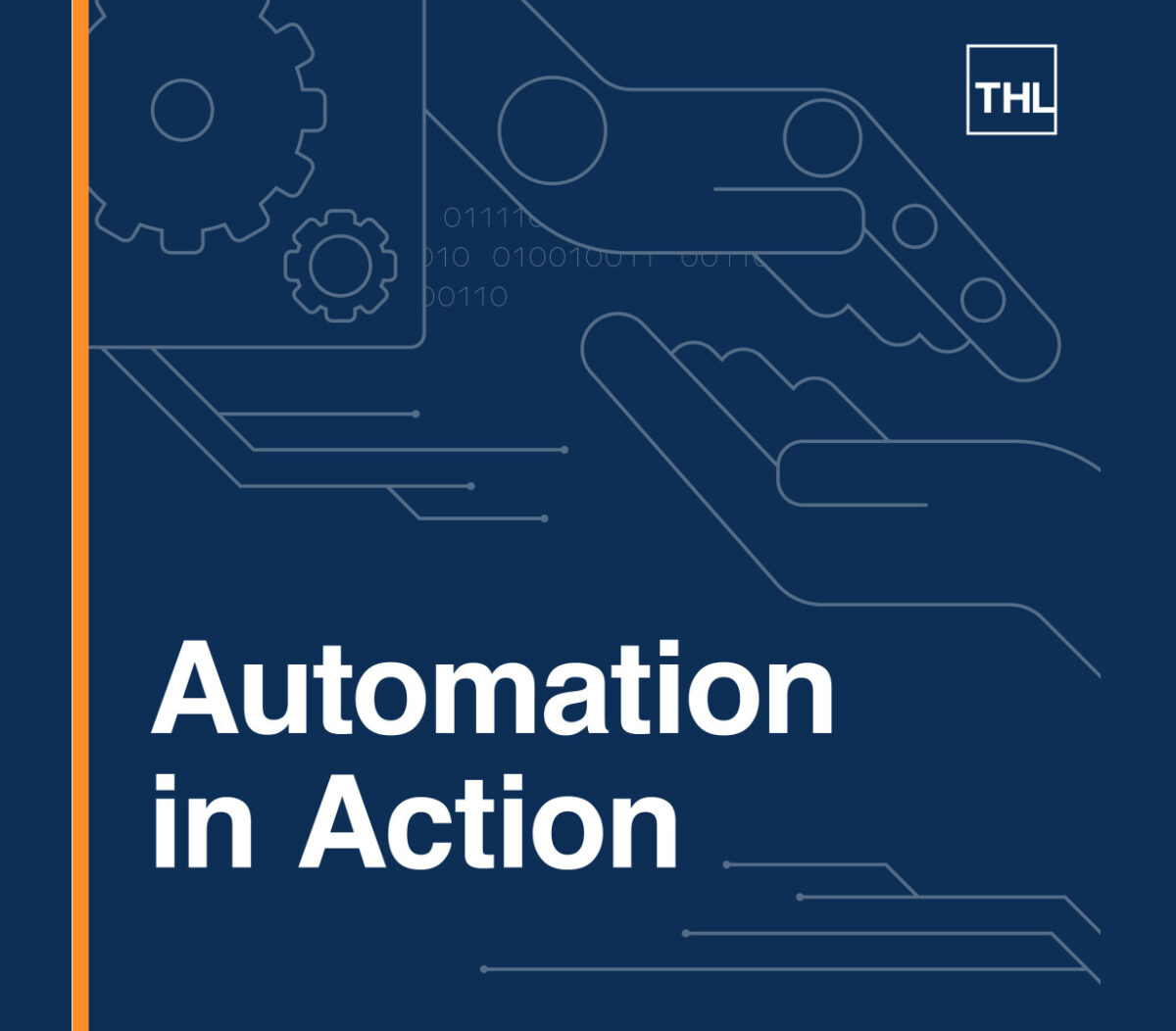
THL’s Automation in Action, Episode 7
At its core, insurance is about risk – how to detect, measure and mitigate it. And advances in automation technology, generally – AI, more specifically – are transforming the insurance industry. Automation is revolutionizing the way insurance companies make use of vast troves of data to power underwriting and processing, deliver greater value to end customers, and bring new cost efficiencies to the broader market. It’s not just important to the insurance sector. It has a profound impact on each and every end market that uses insurance to manage risk.
In this episode, we speak to Max Drucker, CEO of Carpe Data, who has had a front row seat for advancements in insurance technology, spanning his entire career. We learn from Max how the automation of once manual tasks and the application of AI are creating new tools and methods to revolutionize risk management.
Key takeaways from this episode include:
- What key challenges the insurance industry faces today
- How insurance underwriters use data to improve risk assessment and management
- What data availability means for the insurance industry
- How automation is helping to drive the industry forward
- What AI portends for the industry and its clients
To learn more about THL’s cross-sector strategy to uncover opportunities in emerging technologies, visit THL.com/automation.
[00:00:04] Max Drucker Before Freakonomics and Nate Silver and before this entire arena of predicting outcomes was cool. Insurance carriers were doing that from actuarial science perspective. It’s always been really interesting because what they’re really doing is they’re taking historical data and looking to predict what’s going to happen in the future.
[00:00:21] Jim Carlisle That’s Max Drucker, CEO at Carpe Data. And this is Automation in Action, where we pull back the curtain on automation technology and lead you on a journey inside. Once again, I’m joined by Sarika Ramakrishnan, who sat down with Max for an in-depth conversation on insurance automation. I’m also joined by my colleague Ed Shahnasarian who leads THL efforts and insurance technology. Ed, why was Max the right person to talk to, to understand how automation technology is transforming the sector?
[00:00:53] Ed Shahnasarian Thanks, Jim. Max has been in insurance technology effectively his entire career. He started at Apple in 1998 before being recruited to join the first online auto insurance carrier. Believe it or not, that was over 20 years ago. He was part of the technical team that built an insurance carrier from scratch to the coverage. He credits his career for teaching him a lot about insurance, leading him to where he is now the Carpe Data. A lot of people probably assume insurance is a lagging industry in adopting new technology. It’s widely perceived as a legacy sector in a lot of ways, that’s true. But as Max explains in his interview, the insurance industry has been at the forefront of using new scientific methods and tools to assess financial risk for a very long time. One example, travelers integrated AI and machine learning models into claims processes over 15 years ago, a decade plus longer before ChatGPT entered the scene. But the advent of large language models or LLMs something different. It could very well transform the financial risk landscape in a way that helps both customers and agents. It’s definitely going to transform workplace operations at a minimum. That’s why I’m I’m excited that Sarika had a chance to dive into these topics. Sarika, what are your key takeaways?
[00:02:02] Sarika Ramakrishnan Max really does have a storied career in insurance. It was fascinating to hear from him about the inner workings of the insurance industry, and how automation can really transform it moving forward. What really stood out to me was how analog much of the claims processes, even still today. But it takes experts like Max to dig into the process and find opportunities to inject automation and revolutionize the industry.
[00:02:31] Ed Shahnasarian Yeah, Sarika, the US insurance market is absolutely massive. Over 1.5 trillion dollars of written premium across life, health, property and casualty insurance. So the automation of workflow processes is going to have a absolutely massive impact on the space given the size of that [00:02:49] team.The power of AI can be truly transformative here.
[00:02:52] Sarika Ramakrishnan The scale really is staggering. So I found it really interesting to hear about the different verticals within the insurance space and how automation can play a part in so many different ways. By definition, insurance is about measurement and mitigation of risk, and that’s a critical need across financial services. Delving into automation of insurance seems like a logical starting point.
[00:03:18] Ed Shahnasarian I’m ready to hear more. Let’s get started.
[00:03:22] Sarika Ramakrishnan Hi, Max, welcome to Automation in Action. Looking at your career, it sounds like you played a really large role in the digitization of insurance. What is it about the insurance market that interested you in the first place? If you were talking to an early career professional, what would your pitch be?
[00:03:41] Max Drucker I think lots of us are really interested in predictive analytics and, and data science. At some level, predicting the future and insurance careers of predicting outcomes for literally centuries. Before Freakonomics and Nate Silver and before this entire arena of predicting outcomes as cool, insurance carriers we’re doing that from actuarial science perspective. It’s always been really interesting because what they’re really doing is they’re taking historical data and looking to predict what’s going to happen in the future. So I think these are really profound and really interesting challenges. It’s an area in which they need lots of talent. And it’s really interesting problem and really interesting challenges. I think it’s a really great opportunity for ambitious, smart young people to go into it because again, it’s not the obvious choice necessarily, but the problems are profound and super interesting. And obviously the scale and the size is tremendous and the opportunity to to drive impact. I can’t think of industries where you can do more, frankly, from it being able to to move the needle for for entire company or for the entire industry.
[00:04:48] Sarika Ramakrishnan What are the size of these insurance markets?
[00:04:52] Max Drucker In the US, between life and health and property and casualty, looking at roughly 1.5 trillion dollars of written premium, that’s a bit about 50/50 between the two and how you categorize it. But in PNC, which is here and where we’re certainly I’ve had the most experience, seven or 800 billion dollars of written premium annually. So the scale is tremendous. You talk about the number of employees that work in the industry you’re looking at, according to my good friends at ChatGPT. So don’t necessarily quote me on this, but probably count to take and it basically split down to about 600,000 that work in the life and health space, about 900,000 working in PNC, and about another say million, two or so work in the brokerage and agencies and ancillary non-care related businesses. So you’re looking at really big numbers of people that are working in this industry. And obviously we’re here to talk about automation, lots and lots of opportunities to automate across when you have such an incredibly large employee counts.
[00:05:56] Sarika Ramakrishnan Wow, that really is tremendous size and scale as you noted. Seems like the the insurance industry is is fascinating and, solving profound challenges and problems. I think it’d be helpful to dig into some of those key challenges and problems that insurance professionals are working to, to solve everyday. Maybe we can start with assessing risk for for different industries and businesses. And maybe you could walk us through the problems and, and how the insurance industry is working towards solving those.
[00:06:34] Max Drucker Sure. Absolutely. Ultimately, the problem that the insurance carriers are trying to solve, right, is they’re trying to enable whether it’s a person, an individual, or whether it’s a business, to be able to go and live their lives without having the risk of a catastrophic disaster. Right? Life insurance takes care of that. If you’re a business, you can operate in a in a way with some comfort. So the insurance carriers need to solve that problem of how can I enable effectively the customers to do whatever they need to be able to do and not have the devastating downside risk, yet still provide a product that’s affordable enough to for it to make sense. So that’s obviously a big picture problem. But to the individual use cases, it’s just the classic case that we all have of having to make decisions with information. You don’t know what’s going to happen in the future. You don’t have all the pieces of the puzzle. It’s a highly regulated business as well. Certain things that that aren’t automated and that don’t have the right data around it are somewhat subjective. So you don’t have consistency. And so you’re also at the whims of other industry trying things like inflation can be completely outside your control. Interest rates and making money on investment come can be someone outside of your control. So there’s so many variables that are hard to manage. They have to make the best decision they can and they commit to a year out or in the case of life, decades out.
[00:07:55] Sarika Ramakrishnan What are the broad categories of data that inform decision making and risk analysis? And how do insurers try to gain an edge in their underwriting of the various risks that you mentioned?
[00:08:10] Max Drucker It breaks down a lot between the line of business with type of insurance and whether it’s about an individual or for a business, but to to start in that sort of the most basic and in auto insurance, the data elements that the foundational data elements of auto insurance, and this is what ultimately led to the automation of auto insurance is the automated motor vehicle report. Right? A motor vehicle report you’re driving history is very predictive of your future likelihood of getting into an accident. The automation of a loss history report, how if you’ve been in previous accidents, is very predictive of if you’re going to get to a future accident. And then in most states, but not all states, the credit score is another core component of what goes into being able to price and underwrite an issue that policy, because credit is very predictive of loss and insurance outcomes as well. So those are the foundational elements has something like auto insurance, which are really very accessible and automated. And that’s how we’re today largely auto insurance has totally automated. But if you go to something like a large commercial, if you are looking to price and underwrite a Fortune 500 company, for example, there is not a data element off the shelf that you’re going to be able to implement input to a model and issue. What’s really interesting about this time now, and I think what a lots of data companies are thinking about hits okay on auto insurance, where on one side of automation, on large commercial you have almost no automation. But what are all the other processes? How do we move across that spectrum and in areas like homeowner’s insurance? There are some elements that you can find that you can build into that process that you got many around, but there still a lot of manual processes. There’s still a lot of things with home inspections. They’re doing a lot of things with questionnaires that are hard to verify. Ultimately, it’s the operational efficiencies around the manual processes are very costly and ultimately make a bigger challenge for carriers to be able to really scale their businesses.
[00:10:15] Sarika Ramakrishnan Insurance professionals really do predict the future, don’t they?
[00:10:19] Ed Shahnasarian Really does seem that way.
[00:10:20] Ed Shahnasarian And in hearing from Max, it’s clear the industry has made a lot of strides over the years to improve and streamline processes.
[00:10:26] Sarika Ramakrishnan And lead to better business outcomes as well. I was curious, though, especially given that such a large part of this data is built on predictive data. It made me think about the emergence of AI, and how it may be able to make even more improvements to the process. There’s so much data out there that we can learn a lot from.
[00:10:46] Ed Shahnasarian Really looking forward to hearing what Max has to say about that. Let’s hear more from him now.
[00:10:58] Sarika Ramakrishnan It sounds like auto insurance has been an early adopter of automation, and some of the other segments are still catching up. Why was auto insurance an early adopter?
[00:11:10] Max Drucker I think auto insurance is a confluence of, because it’s so highly regulated, the data elements that comprise a policy, a quote are pretty consistent and again, mandated from regulatory perspective. So the 16 or 20 or 25 data elements, such as your years experience of driving, your marital status, your education level, but the driving history is a huge component of it. That’s a consistent area. So the data elements were consistent the cues we’re looking for and we were able to automate that. DMV across the country have made their data available to be accessed via APIs. There is a long history exchange that has made that available to be available via APIs and in credit, of course. It’s something that banks and many other companies use. And so all the things came together at the same time. And that’s why auto insurance has been such an early adopter and has now for more than 25 years, been a largely are their product and where is today effectively 100 percent automated.
[00:12:08] Sarika Ramakrishnan Got it. Where do you see the future of automation for other markets that that are still catching up and don’t have that same level of data availability?
[00:12:19] Max Drucker So if you look at auto on one side and I talked about big large commercial on the other side, say take small commercial and maybe put that somewhere in the middle and initially don’t take it from me, take it from the leaders of the major insurance carriers. Evan Greenberg from Chubb on CNBC said not too long ago that they’re looking to get to their ultimate goal of asking two questions about a business what the name, what the address, and they’ll be able to produce a quote based on the data. That’s the goal. And they said that in their interim step is to get the seven questions. So this is a very consistent theme across small commercial carriers, at least for the leading edge carriers to want to ask a few questions as possible. Today, depending on the carrier and getting the process for a restaurant, for example, sometimes they’ll ask 70 questions, 75 questions, and some of these things are anecdotal and that they just put into a process. Some carriers still apply that it’s more of a an art than a science approach to how they underwrite and how they select and have the right business. But the very clear trend from leadership, from the large carriers is to reduce the questions and have the questions be derived from the available data, from the data providers that are out there. So they can. And that’s why it’s so important for the data providers to always air on the side of precision. The veracity of the data is what makes it possible for that given carrier to be able to then issue that policy. So just to give a small example on the type of approach, the carrier wants to know if there’s a swimming pool at that apartment building. Right? Because that’s a huge risk area. And that’s a that’s really important. Right? They need to understand that. So we talk about technology a lot. And I’m obviously in that, building technology business. And we all talk about all of the functionality. We love talking about AI. We love to talk about computer vision. We love talking about machine learning. We love talking about all of the things that go into the capabalities that are available today that weren’t available maybe 20 years ago or 10 years ago. But to the carrier, they care about the use case, they care about the value. They care about, is a swimming pool there or not? Step one, you want to look at apartments.com and the apartments.com say, “there’s a pool there?” Okay. That’s great. That’s good to know. The structured data element. That’s helpful. Right? Two, maybe I want to look at the website for that apartment building. And maybe the apartment building website is describing the pool. We’re talking about the hours of availability for that pool. So that’s another great indication. But now I can have more confidence in that accuracy in that veracity. But there’s also a model. Maybe I don’t have that data, but I know that apartment buildings on this same side of the street that rent for roughly this amount all have pools. So I can impute and predict that there’s a 92 percent chance there’s a pool here. And so they can work in that. Or I can look at an image from whether it’s their website or whether it’s from Google Images or from whatever that is. And I can see that there is indeed a pool here from that model. And so by taking this multidisciplinary approach, you’re just getting down to is there a swimming pool here, yes or no? I can dig that the bank and I can do that policy. And then that carrier can really move on and really automate. That’s what drives the automation. It’s all of that technology is what unlocks what they need to know from a causal risk perspective to be able to issue that policy.
[00:15:45] Sarika Ramakrishnan That’s super helpful and a really illustrative example. What is the key challenge or the key set of challenges that is getting in the way of going from 70 questions, as you mentioned, to two questions, what is the unlock that your team is working on to to bridge that gap?
[00:16:06] Max Drucker On one side, it’s in the 70 questions you want to really dig into, to rethink some of it and understand how predictive really are. All is is knowing the number of tables in the restaurant that predictive or is a proxy on that, the amount of revenue you’re doing close enough, right? Something like number of tables in a restaurant is a very difficult number for something that even an agent to know, and it will change. Or however, there’s the kind of thing that a carrier historically has really want to know. So step one, is to really understand. What do I necessarily really need here? But what I also want to do the character would be looking at this is what is available data is. This is what’s possible, right? Some things are not going to be possible. It’s going to be very difficult, let’s say to even the current eras of generative AI to surmise the number of tables at the restaurant, right, or the number of people inside that restaurant per hour, whatever those things are, these are hard things to do with any degree of accuracy. But there are lots of other elements that that, again, that you can get at today about that restaurant, things you can learn from the menu on that restaurant. Do they have oysters? They’re all seafood. That’s an area that carriers care about. The things that you can service. Do they have a deep fryer? That’s the kind of thing that carriers what understand about because there’s a potential fire risk. You can get that a lot different ways. One of the ways you can do is you look at the menu. Do they have French fries? Do they have your chicken fingers? Some really looking at okay what’s the available data. And then what can you it’s like proxies for what they historically thought about what was going to be predictive. And that’s how you are able to reduce those number of questions and then enable you to then automate around those questions. So then the lookup becomes name and address and then the rest of it pre-populates and you can have that, then you can understand this is what this business does. Is it a bar? Is it a bowling alley or is it both? We can automate that step. And that’s a really important step. So the classification part so that we can largely do today. And the internet has made that very possible for so many businesses because most businesses are online. Degree of accuracy, they generally will suggest maybe 20, 25 percent of the businesses that they have or small commercial or misclassified. It can be because when they were entered in as a lawyer, it turns out they were a landscaper. And it was a you literally did entry issue or it can be simply the calculation was difficult. The agent didn’t do it right. So it can be a lot of different reasons, but there’s long misclassification. So this is really, I think, what most would agree is the first real pillar of automation is automating that classification step. Right? What does that business really do? Step two is automating around that specific risks but ensuring that the data is accurate. The veracity is there by talking before about taking a multidisciplinary approach. But the third category, this which is so critical and so important, is then select segmenting the business, being able to compare two different businesses that on paper look the same. So I need to be able to compare Joe’s Cafe to Harry’s Cafe because they look the same. But what they do now can tell me what’s a better risk. And what I think is super exciting is there is new data out there that is predictive of loss outcomes that carriers are currently exploiting. In examples of that are the social presence of that given business. The reviews and what people say about a business online actually will tell you quite a lot. A restaurant that has a really bad reputation is indeed more likely to have a loss within 12 months, as a restaurant has a really good reputation, as quantified by a score that we’re able to derive. If that restaurant owner often maybe doesn’t make the effort to do anything to whatever online presence is, that might be an indication they’re not doing other things too, making sure they’re putting out a sign for a wet floor or other given areas. And so it can often be a proxy for how that business is run and how well the business run is a very close predictor of what potential loss might look like.
[00:19:58] Sarika Ramakrishnan That’s fascinating and makes a lot of sense. It sounds like there’s three key elements. One, availability of accurate data. Two, prioritizing the data to find out what’s most predictive. And three, processing the data as we think about the progress that’s been made across those elements and what you just mentioned. How is that progress evolved over, say, the last 30 to to 50 years?
[00:20:31] Max Drucker I think we’ve made a ton of progress, but we have a ton more to go across the board. So in just the last, say, two or three years, it has really been an acceleration, a real push towards more automation. But still, historically, the number of carriers that still rely heavily on paper is very high. Right? Major carriers that we all talk with, they love to run mainframes, and they still do lots of paper, even from our application in tech. Right? There is a manual process somewhere where somebody is inputting it into a system. So there’s still so much of that across so many different aspects of the entire policy lifecycle. Largely when the markets are good, insurers are making a lot of money. They haven’t had the urgency historically, but the urgency is here now. So the insurers are now that inflation has come down a little bit, the business of stabilizing a little bit. They are now understanding and making these investments to make sure that they can scale their businesses. They can do more with the same head count. And as people age out, as people retire it will automate more and more of these traditional processes.
[00:21:35] Sarika Ramakrishnan It sounds like there’s been a lot of progress. But to your point, still a lot of room for further automation. How do you see AI particularly large language models transforming the sector?
[00:21:47] Max Drucker It’s obviously super exciting and something we all talk a lot about. So to take a slightly broader view to that question, I think about it in three separate ways. I think the first way, and I think this applies to the insurance carrier or our customers, but also to take it to a company like MYER or to Chubb or any other tech companies. The first category is how am I using the LLMs or generative AI, but the way I like to describe it is there AI. Right? AI that is clearly a step up then AI a year ago, so how am I using this new AI internally for internal productivity gains? So really across the board internally how as a company are we leveraging this to increase our own internal operational efficiencies on the things that we did that we do traditionally. So I think that’s category one and that’s really important. The second category is how we’re using the LLMs to reduce our cost of goods sold to our product. So whether if it’s an insurance carrier of the cost of goods sold, is whatever their manual processes might be. Right? Obviously they’re cost in those given areas. But to that, if they can automate more of a process because of the LLMs has made that possible, that reduces their costs, which enables them to either make better margin or ultimately what they want to do is reduce costs, reduce losses, and be able to purchase prices so they can expand market share. The third category, which I think is really the most exciting category of where we are, has how can we invent new products that weren’t possible yesterday? Right? Because they weren’t feasible from a high price or a responsiveness or whatever that was, what we can do today. And in insurance, the sky’s the limit, right? How can I predict loss? How can I predict outcome? How can I predict retention? How can I predict how much to pay is always what they’re thinking about every single day? So if I have better prediction methods and LLMs really enable that, they can have a better sense on what’s going to happen into the future, or if they’re not necessarily able to do that, they obviously there’s now a number of companies that are able to really help them do that as well. And that’s and that’s the area that I’d like to spend most of my time says, okay. What can we do now that we couldn’t do yesterday?
[00:24:08] Sarika Ramakrishnan Can we talk about Carpe Data specifically, and how Carpe is helping to address this gap and propelling the future of automation in the insurance industry?
[00:24:20] Max Drucker Obviously, I love talking about about the Carpe Data and the areas that we focus on today as both in claims and in small commercial automation around both those two given areas. The claim side, we automate the review of the online presence of injury claimants. So it may sound pretty narrow, but you know, there’s been lots and lots of injury claims every year, whether that’s a bodily injury claim, whether that’s a worker’s comp claim or disability claim. And there’s lot lots of exposure around those given areas. And we have apply a really easy and cost effective solution to get at that. So that’s what we’re doing claims. And we’re continually chip away at more and more components of the claims lifecycle. Again, it’s a incredibly, in many cases very manual, very laborious, time consuming and expensive process. On the small commercial side, has auto insurance is fully automated and log parcels very little of that. Small commercials begin to get a little bit in between. We are looking to move small commercial, is far towards auto has possible. So we are building these foundational elements to enable that automated decision making, to enable that embedded data where rules and logic can be built around so they can then issue that policy, they can decide, I want to prioritize this good risk and be able to issue this policy. I want to be able to pass those policy through it quickly as possible. Maybe I’m able to even enable them to reduce a rate so they can gain market share and segment that they really like, but we’re also giving them the opportunity to avoid those bad risks. As risks that the data is irrefutable, predictive of a bad outcome or worse outcome than similar type risk. So what we’re able to do for them is give it to them in an easily consumable way, a consistent, structured format with broad coverage to enable that automated decisioning to enable that being embedded in a process. And so they can write as much as they want to, having to look at very large percentage of that and see the quality of their book imprint.
[00:26:19] Sarika Ramakrishnan That sounds like very interesting problems to to solve and exciting from an automation perspective, seeing where the the auto market is and bringing other markets to a similar level of automation. Well, Max, thank you so much for taking the time today. And joining me. This is a really insightful conversation and very exciting to hear about the level of automation and progress in the insurance industry today, and all of the exciting things that Carpe Data is working on to propel automation forward.
[00:26:51] Max Drucker Thanks a lot. It’s super fun.
[00:26:56] Sarika Ramakrishnan Max’s interview really addresses two overlapping issues, automation technology in general and AI more specifically. It’s clear that automation has been occurring for a while, but how quickly do we anticipate that AI is going to transform insurance and potentially other areas financial services.
[00:27:17] Ed Shahnasarian In some cases, in insurance, immediately you think about simple workflows that today have high human touch elements associated with them. Think about issuing a policy and certificate of a policy up front. Right? Today, given data is coming in and insert through different mediums, PDF, email, fax lines and Excel and human beings are aggregating that data created together and actually issuing policies and certificates. That’s something that AI was in disarray mediate immediately. And there’s other use cases. If you think about some of what Max is talked about, right? Big data, how AI unlocks other forms of data. Right? That’s going to take years to reap the benefits of that opportunity exists, but the technology has to transform to to capture that data and actually weaponize it to better underwrite and clear claims.
[00:28:08] Sarika Ramakrishnan Where do you see opportunities to create better insurance markets through better technology?
[00:28:14] Ed Shahnasarian We’re seeing it today. And again, data is a powerful driver of enabling that data parallel technology. Right? You think about the telematics sector. Auto insurance underwriting 10 years ago was based off of a go score, a zip code, some other demographic data type of vehicle that’s being transformed today to tell medics whether it’s a dongle in your car, whether it’s your cell phone acting as a data collection device, and sharing that back to the insurance carrier and in some cases, even the OEM, you’re seeing auto insurance underwriting get massively smarter and better because we can now underwrite the driver’s actual driving capabilities, how defensive they are, how much a risk are they putting themselves or their cars in the vehicle based off the data feed that’s coming to them also potentially benefits consumers. If you’re a good driver safe driver, you can pay lower premiums and reap the benefit of being a better driver and less of a risk to the insurance carrier.
[00:29:09] Sarika Ramakrishnan Have you seen concerns about data privacy and ethics? Given the emerging power of AI to drive change in the industry?
[00:29:17] Ed Shahnasarian Yeah. Data privacy is a huge concern right now, particularly in insurance. Let’s say for a couple of reasons. Maybe first off, fraud rights and the ability to use generative AI to create images that could potentially be used like a claims process, right? I could say, hey, show me a picture of Ed Shahnasarian in a car wreck. That that picture being generated. That picture could spur an insurance claim and then a finding and then cash paid out to an insurer. That’s all fraudulent. Huge concerns around that and the use of individuals names, right? Today, many of the LLMs are precluding folks from creating images that are associated with individual names. Right? So that their privacy is covered today. It’s just a matter of time. At some point you’re going to see you’re going to see that technology of all of those walls come down, you know, individual people’s identities being subject to generative AI and the creation of of images, videos, voice recordings, etc., that could be used to support fraudulent claims submissions. So that’s on the claims side. And I think about the underwriting side as well. Right? AI is going to open up a massive data lake. And so yeah, this is this are state by state regulations or also federal regulations on what you’re allowed to use and not use to underwrite their violations around this in the past from different carriers, different use cases. And a lot of times they don’t even know it. Right? The explosion of content and data access that these carriers are going to have, they’ve got to be very careful.
[00:30:46] Sarika Ramakrishnan As a long time investor in insurance technology and services, you’ve seen the massive evolution of that sector over time. From that, have you seen learnings for other areas of financial services as well?
[00:31:02] Ed Shahnasarian As we think about where we want to put money, the work of this space, how we want to invest behind these trends, we first think about where is the innovation going to come from and what is it going to impact? I think we can all agree the insurance carriers will likely not be the tip of the spear in terms of cutting edge technology and innovators in this space. That’s not what history would tell you. I think these services are going to be vended and outsourced. That creates an investment opportunity for folks like us. And then the question is really, where is it going to be applied and when? I think we see the most near-term opportunity taking place in a lot of the servicing and TPA work associated with everyone from cradle to grave, from issuing policies, policy management, claims management and renewal. Right? Those are the TPA industries, a massive industry for hundreds of thousands of people onshore and offshore that are really collecting and moving paper around because the worlds of insurance is inefficient today. Right? The the automation of that entire workflow feels like the first one to come, and is where we currently have our sights and our priorities set. I think over a longer period of time, you’re going to see insurance software. Think about the core policy admin systems, which are huge systems that are managing complex pieces of data, huge amounts of data benefiting from AI. I think today it’s a little bit more of a question mark on where that fits in. Does that innovation come from that with the policy you have and providers, vendors will see that’s a longer term opportunity that we’ve got our eye on. And we’re focused on insurance today where AI certainly making an impact. But we shouldn’t minimize the broader impact on the financial services industry. We’re seeing across areas that we spend time in, our ISOS. Think about think technology, which is extremely analogous to insurance. So where the opportunities are being felt there, but also across our other areas of focus, trying to think about wealth and fund, CFO software with embedded finance. We’re filling in across the board in AI is extremely topical across multiple services right now.
[00:33:01] Sarika Ramakrishnan Ed, thank you so much for sharing your insights. Now back to Jim.
[00:33:05] Jim Carlisle I’d like to extend a huge thanks to Max for joining us on the show, to my co-host Sarika Ramakrishnan and to Ed Shahnasarian. Thank you all for listening to Automation in Action. Automation in Action is brought to you by THL. To learn more about THL cross-sector strategy to uncover opportunities in emerging technologies, visit Thl.com/Automation.
- Verticals
- Financial Technology & Services (FTS)
- ISO
- Insurance
- Cross-Sector Strategy
- Automation