Podcast
Human in the Loop: How Generative AI is Transforming Knowledge Work
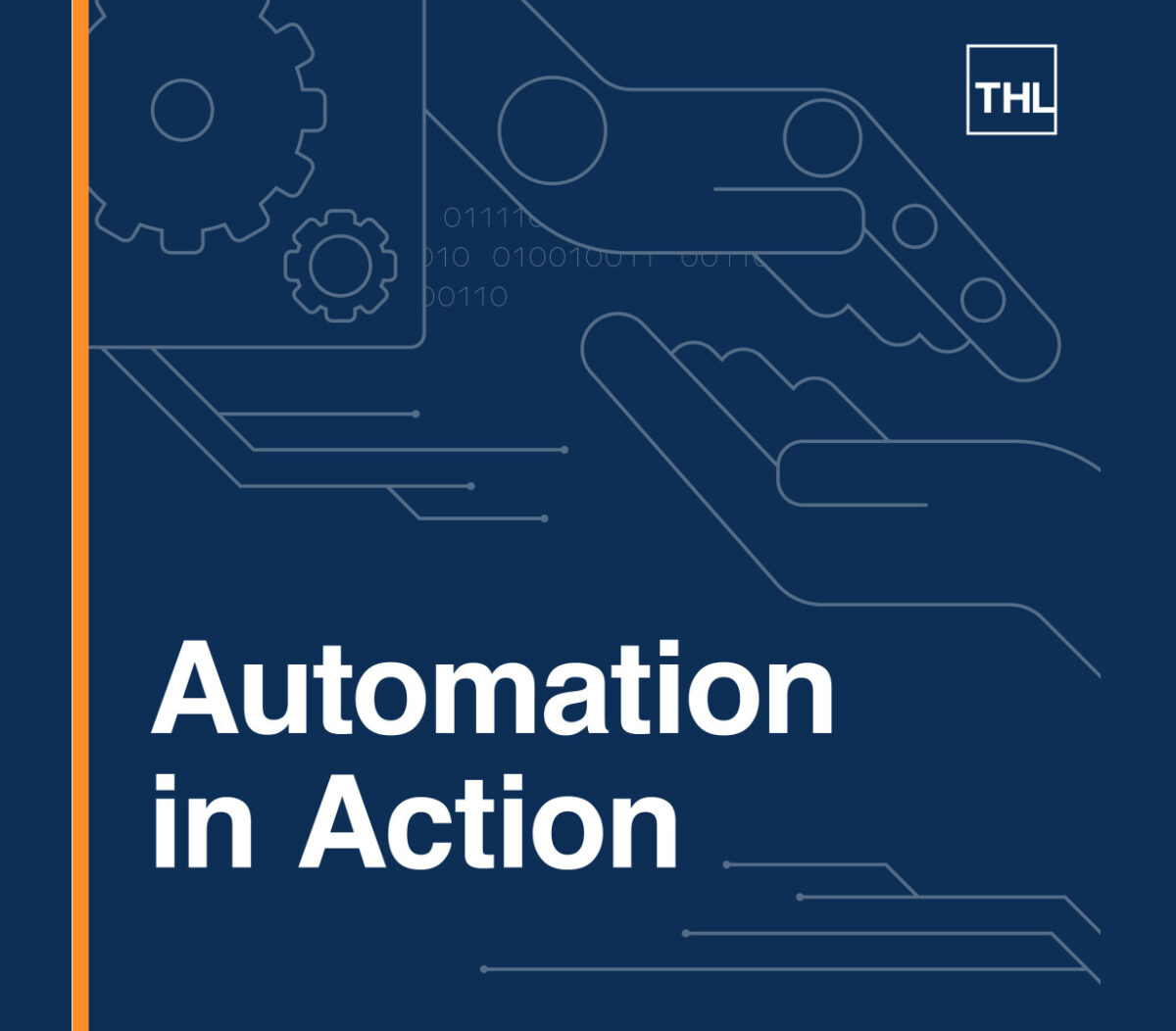
THL’s Automation in Action, Episode 4
The upsurge of generative AI has reached an inflection point, with the potential to revolutionize industries as diverse as marketing and communications, accounting, securities trading, advertising, but also coding and computer science. It’s an exciting future in which non-technologists can use AI to build powerful applications that will transform their industries, but it’s also a disruptive world that may change the role of millions of knowledge workers. In this episode, we speak to Dan Roth, Co-Founder and CEO of Scaled Cognition. Together, we’ll take an in-depth look at how we interact with these emerging technologies, the future of generative AI, and what we need to do to prepare for it.
Key takeaways from this episode:
- The inner workings of large language models like Chat GPT, and how they learn and adapt.
- The forthcoming impacts of emerging technologies on knowledge workers.
- How these emerging technologies will affect the way humans interact with computers.
- What business leaders need to be aware of when engaging with new technologies.
To learn more about THL’s cross-sector strategy to uncover opportunities in emerging technologies, visit THL.com/automation.
Dan Roth [00:00:04] The industry has steadily evolved for many, many years, but I think most folks are sort of familiar with the exponential curve that most advanced technologies follow. And I think we’re just on to a steeper part of that curve right now. I do think that there are a lot of lessons from the past which still apply. Certainly the last five years have been been very exciting with a lot of incredible innovation, certainly in language processing.
Jim Carlisle [00:00:32] That’s Dan Roth, co-founder and CEO of Scaled Cognition and former corporate vice president of AI for Conversational Interfaces at Microsoft. I’m Jim Carlisle, and this is Automation in Action, where we pull back the curtain on automation technology and lead you on a journey inside. I’m here with my colleague Alex Sabel, who had the opportunity to speak to Dan about generative AI and how we interact with these emerging technologies and what the future looks like. Thanks for joining me, Alex.
Alex Sabel [00:00:59] Great to be here, Jim.
Jim Carlisle [00:01:01] When the conversation turns to generative AI, as it often does these days, we start to think about massive change on a global scale. The industrial revolution would be one example. The Internet is another. This time we’re really, really cognizant that the amount of innovation that’s that’s coming at us with generative AI is going to have a massive impact to humanity. And in order to prepare ourselves for that, in order to understand the impact on the economy and where our world is headed, we need to understand the technology, its potential, how we can harness that potential in a way that delivers both broad benefits to the economy and to society at large. Alex, what was your biggest takeaway from your conversation with Dan?
Alex Sabel [00:01:45] What really struck me was speaking about productivity. And it’s interesting you bring up the industrial revolution because there was actually an essay published in the 1930s by John Maynard Keynes titled Economic Possibilities for Our Grandchildren, where he infamously predicted the 15 hour workweek 100 years later. Clearly, that never happened because consumption didn’t stay content and people wanted to consume more things over time. So it was really interesting to hear from Dan the way that as our productivity increases, the desire for greater output increases almost concurrently. It’s not that I will necessarily reduce labor or labor hours. It’s more the case that augment what we do with those labor hours.
Jim Carlisle [00:02:21] Yeah, I think that’s exactly right, Alex. None of us are working 15 hour workweek. Maybe we wish that we were, but we’re certainly not. And we’ve seen massive shifts in productivity as a result of the investment in technology. Are we ready to hear from Dan?
Alex Sabel [00:02:36] Let’s get into it. Dan, great to speak again.
Dan Roth [00:02:39] Yes, great to be here.
Alex Sabel [00:02:41] Can you help us understand how the field has evolved since you first started, you know, 20 plus years ago?
Dan Roth [00:02:47] I mean, I think some of the most profound changes have really occurred in the last five years, particularly with transformer models becoming refined enough to to really become useful. The timeframe before that was characterized by much more application specific kinds of approaches. You would have systems for speech recognition or systems for speech synthesis or systems for machine translation, things of that nature. Spam filtering. And I think the thing that’s so interesting about what’s happening now is that you have these much more general pre-trained models which have very broad capabilities and are demonstrating a very important capability, which we just refer to as generalization, which is the ability to transfer learnings from one particular domain to another that it may not have been explicitly trained for. So, you know, the industry has sort of steadily evolved for for many, many years. But I think most folks are sort of familiar with the exponential curve that most advanced technologies sort of follow. And I think we’re just on to a steeper part of that curve right now. I do think that there are a lot of lessons from the past which which still apply. Certainly the last five years have been been very exciting with a lot of incredible innovation, certainly in language processing.
Alex Sabel [00:04:07] Could you help the listeners bridge the gap between what they see happening with ChatGPT or some of their other favorite applications that are starting to implement some of these gen AI powered capabilities and some of the developments happening behind the scenes? How does ChatGPT do it? How do these applications take natural language queries and return text back to the user in a way that the listeners might be able to understand if they are trafficking in this every single day.
Dan Roth [00:04:38] At a very high level these language models essentially predict sequences of words for sequences of tokens. So given training or given exposure to vast amounts of word sequences, and in the case of the language models that most people are familiar with, like GPT-4. These are more or less been trained on all of the text of the Internet. You have these systems which are either weakly supervised or unsupervised and learned simply by observing these vast amounts of data. And what they’re really good at is figuring out, given a sequence of words or a sequence of code or a sequence of a mathematical equation, What would make the most sense probabilistically next? And I think what’s very interesting about them is that they are able to actually creatively solve problems in order to satisfy their purpose, which is to essentially populate what the next best set of tokens or words are. What’s I think really fascinating is that given, let’s say, a math problem that it may have never seen before and seeing that, okay, that the next most probable token in the sequence needs to be, let’s say, a solution to this problem. It can actually solve the problem in order to come up with that token and to populate it, which is really pretty fascinating. That’s without having explicitly trained the system how to, let’s say, solve mathematical problems. This really draws upon their ability to blend knowledge that they’ve extracted from all these various contexts in order to create very plausible, seemingly human language, given a very small prompt from the user. At a high level they’re really just sort of prediction engines. But I think what’s so fascinating and profound about what’s happening now is that they’re actually able to do reasoning or meta computation or order out come up with these next token sequences should be.
Alex Sabel [00:06:44] That’s a great explanation. How does the emergence of these large language models affect how humans interact with computers and more specifically, the software that they’ve historically been used to working with?
Dan Roth [00:06:57] Yeah, this is a really, I think, important question. And I think one of the challenges that industry is going to face over the next few years is coming to terms with what these systems really can and can’t do. The excitement’s in the press and in the business community about the emergence of these new models I think is warranted in certain kinds of applications and is probably premature in many others, particularly those that have to do with automating workflows and software tasks. So it’s going to take a little bit of time for reality to set in. And I think there’s a couple of interesting ways of looking at it. I mean, one is that the opportunity for true automation, I think is still in the future and is probably significantly larger than what we’re seeing today in some of these generative applications. But that said, there are still a lot of workflow oriented tasks that are more content creation oriented in nature. So the kinds of applications the product market fit for LLMs, particularly today, seems to be places where the person is required to either, let’s say, transform data from one format into another or to actually produce new creative content. So you can think of that as marketing literature, you can think of that as producing slides, can think of that even as producing software. These are creative endeavors that are obviously extremely important, and many industries and many processes within most companies rely on those things. And so these are things that the systems today are sort of remarkably good at and I think uniformly require a human in the loop in order to be trustworthy. They’re still very, very error prone and they’re not trustworthy on their own. So these kinds of applications where you might generate a document still require the human being to review the output and ensure that it’s appropriate, accurate, grounded in truth, etc.. If you think about a very simplistic stack of a kind of prompt plus an LLM are really, I would say, wholly unreliable for most mission critical enterprise workflows. So this automation area, I think is still ahead of us and really will depend on the emergence of much more sophisticated tech stocks being developed around LLMs or other kinds of foundation models in order to make them truly trustworthy, controllable and predictable. And that’s to say nothing of some of the unit economic issues, which I think are really still very much an issue for for most enterprises who have any scale. These systems are still very expensive to run at inference time. The latencies are still, I would say, unacceptably long for anything that requires an interaction with a customer for most things. So I would say we’re very early in the process, but we definitely are, I think, getting a glimpse of the future where this technology has the potential to democratize computing by really lowering the expertise that the human beings need in order to be able to exercise rather sophisticated functionality. Automation is coming, but kind of put a little bit of context around that most people have heard about some of the failure modes of these large systems, including things like hallucination, which is not the end of the world if it’s drafting an email for you and you can go in and correct the mistake. But if the system is driving an API to a backend system and hallucinates a variety of API calls, that can have disastrous consequences. So, you know, we’re really not at a point yet where I think we’re looking at very large scale automation, but I think it’s on the horizon.
Alex Sabel [00:11:10] Dan made a couple of really good points here that I think we should emphasize the first of which being computer human interaction. If we look back to the first computer, it weighed 30 tons and took up an entire room. You could only perform one problem at a time, had no operating system. Even mapping a new problem on to the machine could take weeks because of all the switches and cables that had to be manually adjusted after significant advances in the preceding years. We eventually got command line interfaces which allowed users to interact with the computer through lines of text with the help of keyboard and terminals. The issues were these interfaces required specialized knowledge, no room for error and the actions performed were irreversible. Then, as graphical user interfaces commonly known as [00:11:52]GUI’s emerged, [0.5s] it allowed users to interact with the computer programs through icons or visuals instead of text based interfaces. Equipped with a mouse, the addressable market that could now operate a computer was expanded to millions, basically anyone with fine motor skills. Obviously we’ve seen massive improvements in hardware, software and user interfaces over the past decade, especially in the devices we carry around in our pockets every day. So I generally view the ability to interact with computers through pure natural language as another step change in how humans interact with computers and a quicker, more intelligent way, potentially further democratizing the ability to access information or even outsource intelligence.
Jim Carlisle [00:12:31] Yeah, Alex, that’s that’s a great point. There’s a lot of big words in what you just said, but to simplify it, there’s a real technical barrier for lots of ordinary business users to access information. You know, I have to call my IT guy and get him to figure out how to plug all this stuff together. It seems like some of that’s changing. You know, the way that knowledge workers are interacting with machines has a potential to really change because business users can use normal business language to reach technical outcomes.
Alex Sabel [00:13:02] And that’s exactly where Dan and I go next, the ways in which knowledge workers will interact with AI. As you think about the benefits that these large language models bring, how do you see the large language models affecting the future of work, more specifically, the future of knowledge based work?
Dan Roth [00:13:21] I think that technology has proven to make people more productive, but not less busy. I think it’s really been shown that the expectations for productivity don’t drop. And so as more advanced tools become available, I think the expectation for hyper productivity just continues to increase. So I certainly don’t see LLMs or really any technology making life easier or less hectic for knowledge workers.
Alex Sabel [00:13:57] How might the average knowledge worker benefit? Where does the value accrue to the end user?
Dan Roth [00:14:02] I think that it may change the nature of knowledge work. I think the particular tasks that are expected knowledge workers may change as some are more suitable for automated or generative systems, but I think then their roles will become more focused on managing large scale fleets of these systems that are force multipliers. You know, certainly in in creative pursuits, the production of a sort of first draft of various things is going to be made much, much easier. So if you’re producing a report or a document, an email, an image. I think that these systems are going to make it possible for people to iterate much more quickly and explore a much wider range of different concepts that they may want to continue to pursue. I think that initial step of trying to ideate I think is going to be accelerated and probably greatly enhanced.
Alex Sabel [00:15:01] I want to return to a point that you made earlier on the foundation models. There’s obviously a proliferation of these models emerging and you’re seeing in the market a divergence between massive domain adaptable models and then also smaller specialized models that might be able to run on localized hardware. How do you view the divergence between these two subsets and also the proliferation of endless amounts of closed and open source models?
Dan Roth [00:15:33] We’re certainly seeing a variety of models emerge that have different hardware requirements, can see the seven billion parameter models and all the way up to the very largest ones, and they have different requirements for trading and inference. Generally speaking, many of them are still pre-trained on public, loosely internet related data. And then to the extent that they’re being directed at a particular task, they’re generally being fine tuned. I think that it’s probably going to be the case that open source models are able to keep pace with or entirely catch up with some of these higher performing very, very broad models in the near future, especially to the extent that the data sources for both remain the same. So to the extent that very large systems that GPT-4 is of the world are being trained on the Internet, even if they’re becoming multimodal and starting to ingest things like video and image data, I mean even to the extent that they become models that synthesize the output of several different models. You know, I think that we’re going to see that the open source community keep up with that pretty well. I also think that over time, the computation that’s necessary for one of these models to be at a performance level that’s useful is going to drop dramatically. I think that the very largest technologies of the day will still consume all of the available compute and all of the available data.
Alex Sabel [00:17:09] It’s amazing to see the pace of innovation, especially as it relates to the training architecture and how to best influence some of these models.
Dan Roth [00:17:16] There is a tremendous amount of effort going into each of those things today. It’s not lost on anybody how just astronomically expensive these models are, both in terms of electricity, their carbon footprint, the amount of water they consume, the heat that’s produced. I mean, these are massively, massively wasteful models. And when you look at some of the neural activation maps that some researchers have produced, you can see at inference time a very small fraction of the sort of neural layers are actually activated in order to produce a bunch of the answers and responses that that are needed. So it’s unclear how to create an architecture today that will be more efficient, but it is clear that it’s possible. I expect that to happen. And, you know, within all of the big tech companies and certainly within academia, there’s an awful lot of effort going in that direction. And I suspect that they’re going to be quite successful.
Alex Sabel [00:18:22] Dan, I’d be remiss if I didn’t ask. Obviously, the big news lately has been the shortage of compute. How do you see this unfolding in the future? Is this tech cycle reminiscent of cloud in the early 2010s, or is it more like servers in the early 2000 or even telco fiber in the 1990s?
Dan Roth [00:18:38] I think it’s very, very likely that new architectures that are massively more efficient. I think the likelihood of them being developed in the near term is fairly high. I think that probably there is going to be some equilibrium established in the not too distant future for a lot of the requirements. That said, I still see the kind of tip of the technology spear, if you will, outstripping the compute that is kind of available at any one time. So I expect that the most cutting edge research I think is still going to be slowed by available compute. But I think that in the marketplace for sort of the real world applications, I think that things are going to establish a sort of equilibrium because it’s it’s just not going to be necessary to have huge a100 data centers running everything. I think that new architectures that are more efficient than the transformer will be developed and for probably the vast majority of applications, those new architectures will be plenty sufficient. Even if it turns out that from a performance standpoint, they’re slightly less good than, let’s say, today’s best models, but are a thousand times more efficient.
Alex Sabel [00:19:59] If you look to the company specific narrative, you can bucket some of these companies at the application layer in terms of being AI led, meaning their core product is AI and was since inception. And then some of these companies that are AI enabled, meaning their core product historically was not AI, but they’ve been enhanced with the addition of some of these capabilities. How do you see some of these business models emerging? What differentiates a company competing in either one of these buckets? And then do you think there are any characteristics that create an enduring, sustainable advantage at the application layer at this point in time or going forward?
Dan Roth [00:20:41] I think in the short term, the sustainable advantages will most likely be in the area of proprietary data. Think that the sort of traditional moats that exist around companies and industries I think are going to be they may change slightly, but I think that they’ll be fairly durable for next few years and say, next maybe five years. Technology that we’ve been discussing is not so powerful today that it can completely upend industries and I think create a totally new set of market leaders. I think that what we’re going to see is that market leading companies will continue to lead and they will simply do it more efficiently as well as will their competitors by applying this technology in places where there is reasonable technology product fit. There is a kind of misunderstanding that you can take an LLM in a prompt and solve a problem. And it’s true that you can create a prototype quickly, but that there’s a very long road between a prototype and an actual product, a reliable product or a scalable product. So I think, I am of the opinion that that incumbents with differentiation today strategic advantages today, proprietary data today, I think that they’re going to be fine. I think that they need to apply this technology in a cautious and speedy way. But I don’t think I don’t think it’s going to dramatically change the dynamics because I think it’s sort of going to be applied uniformly across wireless industries.
Alex Sabel [00:22:17] As someone who’s obviously been a successful operator in the space over many years, what recommendations, if any, do you have for other operators thinking about potentially experimenting with some of these new capabilities, whether it be on the product side or the more operational side?
Dan Roth [00:22:35] I think the first thing that I would caution my CEO peer group about is to be skeptical about the demos and prototypes that you’ll see. It is really impressive and exciting how quickly you can spin up a demo or a prototype using these technologies today because you can essentially skip big phases a historical machine learning of the historical machine learning development cycle. So for example, the data collection and labeling and training and all of these kinds of things that you would normally take a really long time just to see any signs of life you skip right over are using pre-trained models and they actually can generalize better than those old systems could anyway. And so it’s really, really easy to produce a demo, which is shockingly good and just seems like it’s going to change absolutely everything. But the caution that I would that I would have is that these tend to be cherry picked examples. You really have to get a deep level of familiarity with the way these systems perform in a wide variety of contexts in order to get a true sense of their performance. So it is really going to take time and discipline to figure out from your prototyping efforts which pieces are actually going to be reliable and scalable and deployable, and then just be patient and understand that everyone is on the exact same curve. We’re not seeing a massive explosion of industry disrupting applications yet, despite all of the excitements and hype that surrounds these things, there are certainly examples of really interesting applications for generating marketing content and for manipulating images.
Alex Sabel [00:24:29] The last one from me is a two parter. What keeps you up at night as it relates to generative AI, whether it be ethical, scientific, existential and then on the flip side, what are you the most optimistic about?
Dan Roth [00:24:48] It’s probably the case that we’re now on a path to be able to create technologies which could have societally detrimental impacts. And, you know, you don’t have to go as far as thinking about the sort of dystopian future where these AI are taken, the decision to exterminate humanity. I think that even thinking about our political discourse, the influence of what’s on social media in terms of changing the voting habits of the electorate, misinformation, things of that nature, I think in the short term, we’re kind of already in that war. Actually, when you look at some of the capabilities of these systems with image generation. On the flip side, I think that it also has the potential to have some profoundly positive impacts in terms of thinking about how these systems might accelerate the discovery of new medicines, improving diagnostics in the health care area. I think they have the potential to really unleash the creativity, the positive creativity of people. We’re going to see probably an era of really exciting and rapid innovation in lots of different industries that has been made possible by these kinds of systems and the systems that we’ll will be developing over the next several years. So, you know, I think I think there’s a lot of really exciting, good stuff, but it’s certainly got a dark side that needs to be watched carefully.
Alex Sabel [00:26:23] Dan, I just want to thank you for the time today. This was an incredible conversation. Really appreciate your perspective on this exciting field.
Dan Roth [00:26:31] It’s been my pleasure. It was a great conversation. Enjoyed chatting.
Jim Carlisle [00:26:40] Alex, Dan, thank you so much. I think that was a fantastic conversation. You know, Alex, as we’ve talked about it, I always am really fascinated, excited about the theoretical, the possibilities that can exist as we adopt these new technologies. And then I’m led to wonder, okay, what are companies doing in real life today with this technology? How many people are just using this, you know, ChatGPT toy to plan their next trip to Barcelona or something like that? And how many people are using it for real business outcomes? What do you finding?
Alex Sabel [00:27:13] It’s a great question, Jim. We’re seeing a lot of this innovation in our portfolio in real time in both product facing and operational deployments. When we brought our portfolio company CEOs together recently to share progress, learnings and best practices, we’re really encouraged to hear some of the interesting implementations. For example, we had one company using gen AI to generate job descriptions. They’re a large, very technical company, so this was a huge timesaver. The same company is assessing a legal assistant to review, update and summarize its legal documents too. Another company is implementing a copilot type featured interact with its supply chain visibility platform and even planning to use LLMs to help ingest data more effectively. Another company is implementing an AI first context driven search for digital assets. As you can tell, a lot of these deployments fit across the spectrum of use cases, and there’s definitely not a one size fits all. As the tech evolves, we plan to continue to bring everyone together periodically. We’re all learning at the same time.
Jim Carlisle [00:28:11] Yeah, and I think that all learning at the same time, the last thing you said is something everybody has to remember. I think early on with the early releases of LLMs, we started to see some real concerns from companies that were releasing private information into the public domain. And you mentioned LLMs potentially being used to help with some of that data ingestion problem. I know you led an initiative at our own firm to try to figure out how THL could use an LLM to better understand the data that we all collect as we’re making investment decisions. Maybe our audience would appreciate some of the lessons learned from that effort.
Alex Sabel [00:28:51] Absolutely, Jeff. So we’ve been exploring ways to introduce generative AI to THL workflows and in being one of the oldest private equity firms. We have troves of proprietary data related deals in market research dating back decades. So in the simplest sense, it came down to how can we operationalize our data in institutional knowledge with a large language model to do our jobs better while still early and there are some relevant learnings. Broadly speaking, a good data strategy which I typically define as how data is stored, structured, organized and maintained is incredibly important to the success of the output, especially when you need to give the models additional context outside of their base training. Secondly, there’s a spectrum of generative air use cases. We likely won’t know the full extent of their capabilities for some time, but large language models cannot solve everything. As Dan mentioned, there are certain tasks that alums in their current state are not well-suited to perform. Hype caused me to forget about more traditional AI and machine learning capabilities, which may be perfectly suitable for a task. When you’re holding a hammer, everything looks like a nail and some things just need a screwdriver. Again, these capabilities may become intertwined in the future, but I think it’s important to be able to crawl before running on the technology side. There is no one right solution in deploying these models isn’t even as straightforward as many are led to believe. For example, there are hundreds of open and closed source models available, so selecting one requires a thorough understanding of the use case, strengths and weaknesses of each model. Our model required handling potentially sensitive data, so we had to be mindful of the security architecture to avoid some data leakage. Lastly, inference costs can skyrocket if users don’t optimize. Understanding how cost-scale as more models are deployed in enterprise environments will be important for us as practitioners and investors. For example, if you’re using a closed source model provider, you may be beholden to their pricing. Well, if you’re hosting a model yourself with bought or rented hardware, utilization matters. So I think it really comes down to understanding the objectives of the use case and really implementing enough resources and time behind developing the right plan. A lot of people think that these problems will be solved overnight, but often it will take months, even quarters, to try to get simple use cases into production. Like I said before, we’re all learning at the same time, and I’m skeptical of anybody that calls himself an expert at this point in time. There’ll be a lot of learnings to go in the next couple of quarters from us as well.
Jim Carlisle [00:31:12] Yeah, Alex, I think you’re getting close to being able to call yourself an expert, so don’t don’t sell yourself short. You know, one thing you said was that there’s 200 models or something like that, maybe more that are now available and in the public domain for people to start to experiment with. I remember reading an interesting article, I think, in the Harvard Business Review, if I remember correctly about that. To summarize, competitive advantage being created not necessarily by the LLM itself, but rather by the organization and transformation of a company’s data to be able to use in parallel with this new technology tool. I don’t remember the exact quote, but that was the gist of it. And I think that’s another really interesting learning maybe for our own portfolio companies and companies that are experimenting with this technology is figure out your data first and then, you know, second, figure out how to use that in parallel with some of the new technology tools that have evolved that we’re really taking advantage of across our portfolio today.
Alex Sabel [00:32:11] Absolutely. I think it’s going to require people being very introspective into the process, the data and the architecture that they have internally and potentially fixing some of the gaps in that as they try to get these large language models into production. It’s a very exciting time.
Jim Carlisle [00:32:26] Well, this is a conversation that we could probably have for another two hours. I’m not sure we have time on on this particular podcast, but it’s been fun to hear from Dan. It’s been fantastic to have you lead this interview with him. And so I appreciate both of your time and energy on this podcast. A lot of things changing. It’s a dynamic time to be sure and a very exciting one. So thanks again.
Alex Sabel [00:32:45] My pleasure, Jim.
Jim Carlisle [00:32:48] Automation in Action is brought to you by THL. To learn more about THL’s cross-sector strategy to uncover opportunities and emerging technologies, visit THL.com/automation.